use case
What is a use case?
A use case is a methodology used in system analysis to identify, clarify and organize system requirements. The use case is made up of a set of possible sequences of interactions between systems and users in a particular environment and related to a particular goal. The method creates a document that describes all the steps taken by a user to complete an activity.
Business analysts are typically responsible for writing use cases and they are employed during several stages of software development, such as planning system requirements, validating design, testing software and creating an outline for online help and user manuals. A use case document can help the development team identify and understand where errors may occur during a transaction so they can resolve them.
Every use case contains three essential elements:
- The actor. The system user -- this can be a single person or a group of people interacting with the process.
- The goal. The final successful outcome that completes the process.
- The system. The process and steps taken to reach the end goal, including the necessary functional requirements and their anticipated behaviors.
Characteristics of use case
Use cases describe the functional requirements of a system from the end user's perspective, creating a goal-focused sequence of events that is easy for users and developers to follow. A complete use case will include one main or basic flow and various alternate flows. The alternate flow -- also known as an extending use case -- describes normal variations to the basic flow as well as unusual situations.
A use case should:
- Organize functional requirements.
- Model the goals of system/actor interactions.
- Record paths -- called scenarios-- from trigger events to goals.
- Describe one main flow of events and various alternate flows.
- Be multilevel, so that one use case can use the functionality of another one.
How to write a use case
There are two different types of use cases: business use cases and system use cases.
A business use case is a more abstract description that's written in a technology-agnostic way, referring only to the business process being described and the actors that are involved in the activity. A business use case identifies the sequence of actions that the business needs to perform to provide a meaningful, observable result to the end user.
On the other hand, a system use case is written with more detail than a business use case, referring to the specific processes that must happen in various parts of the system to reach the final user goal. A system use case diagram will detail functional specifications, including dependencies, necessary internal supporting features and optional internal features.
When writing a use case, the writer should consider design scope to identify all elements that lie within and outside the boundaries of the processes. Anything essential to the use case that lies outside its boundaries should be indicated with a supporting actor or by another use case. The design scope can be a specific system, a subsystem or the entire enterprise. Use cases that describe business processes are typically of the enterprise scope.
As mentioned, the three basic elements that make up a use case are actors, the system and the goal. Other additional elements to consider when writing a use case include:
- Stakeholders, or anybody with an interest or investment in how the system performs.
- Preconditions, or the elements that must be true before a use case can occur.
- Triggers, or the events that cause the use case to begin.
- Post-conditions, or what the system should have completed by the end of the steps.
Developers write use cases in narrative language, describing the functional requirements of a system from the end user's perspective. They can also create a use case diagram using a unified modeling language, with each step represented by its name in an oval; each actor represented by a stick figure with their name written below; each action indicated by a line between the actor and step; and the system boundaries indicated by a rectangle around the use case.
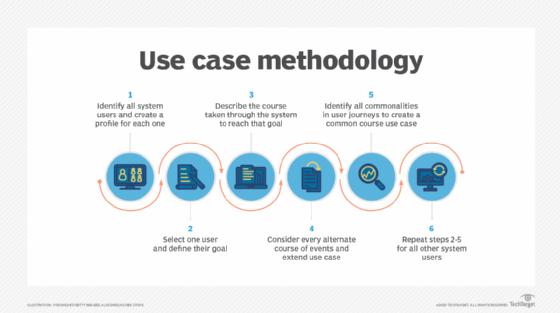
The writing process includes:
- Identifying all system users and creating a profile for each one. This includes every role played by a user who interacts with the system.
- Selecting one user and defining their goal -- or what the user hopes to accomplish by interacting with the system. Each of these goals becomes a use case.
- Describing the course taken for each use case through the system to reach that goal.
- Considering every alternate course of events and extending use cases -- or the different courses that can be taken to reach the goal.
- Identifying commonalities in journeys to create common course use cases and write descriptions of each.
- Repeating steps two through five for all other system users.
When writing a use case, developers may use a sequence diagram -- which shows how objects react along a timeline -- to model the interactions between objects in a single use case. Sequence diagrams allow developers to see how each part of the system interacts with others to perform a specific function as well as the order in which these interactions occur.
Benefits of use case
A single use case can benefit developers by revealing how a system should behave while also helping identify any errors that could arise in the process.
Other benefits of use case development include:
- The list of goals created in the use case writing process can be used to establish the complexity and cost of the system.
- By focusing both on the user and the system, real system needs can be identified earlier in the design process.
- Since use cases are written primarily in a narrative language, they are easily understood by stakeholders, including customers, users and executives -- not just by developers and testers.
- The creation of extending use cases and the identification of exceptions to successful use case scenarios saves developers time by making it easier to define subtle system requirements.
- By identifying system boundaries in the design scope of the use case, developers can avoid scope creep.
- Premature design can be avoided by focusing on what the system should do rather than how it should do it.
In addition, use cases can be easily transformed into test cases by mapping the common course and alternate courses and gathering test data for each of the scenarios. These functional test cases will help the development team ensure all functional requirements of the system are included in the test plan.
Furthermore, use cases can be used in various other areas of software development, including project planning, user documentation and test case definitions. Use cases can also be used as a planning tool for iterative development.
Use case vs. user story
While both a use case and a user story are used to identify system users and describe their goals, the two terms are not interchangeable; they serve different purposes. While a use case is more specific and looks directly at how a system will act, a user story is an Agile development technique that focuses on the result of the activities and the benefit of the process being described.
More specifically, the use case -- often written as a document -- describes a set of interactions that occur between a system and an actor to reach a goal. The user story will describe what the user will do when they interact with the system, focusing on the benefit derived from performing a specific activity.
In addition, user stories are often less documented than use cases and deliberately neglect important details. User stories are primarily used to create conversations by asking questions during scrum meetings, whereas use cases are used by developers and testers to understand all the steps a system must take to satisfy a user's request.
Examples of use case
Outside of software and systems development, another example of a use case is driving directions.
A driver is looking to get from Boston to New York City. In this scenario, the actor is the driver, the goal is getting to New York and the system is the network of roads and highways they will take to get there. There is likely one common route that drivers take between Boston and New York -- this is the common course use case. However, there are various detours the driver can take that will still eventually lead to New York City. These different routes are the extending use cases. The goal of the driving directions is to identify each turn the driver must take to reach their destination.
More specifically related to software and system development, a use case can be used to identify how a customer completes an order through an online retailer. First, developers must name the use case and identify the actors. In this scenario, the use case will be called "complete purchase" and the actors are:
- the customer;
- the order fulfillment system; and
- the billing system.
Next, the triggers, preconditions and post-conditions are defined. In this case, the trigger is the customer indicating that they would like to purchase their selected products. The precondition for the use case is the customer selecting the items they eventually want to buy. The post-conditions include the order being placed; the customer receiving a tracking ID for their order; and the customer receiving an estimated delivery date for their order.
Once the title, actors, triggers, preconditions and post-conditions are identified, the basic flow -- or common course use case -- can be outlined. This starts with the customer indicating they want to make a purchase and ending with the customer exiting the system after their order has been confirmed. After the normal flow is written, all extending use cases should be detailed, recorded and included in the document.
Editor's note: This article was written by Kate Brush in 2020. TechTarget editors revised it in 2022 to improve the reader experience.